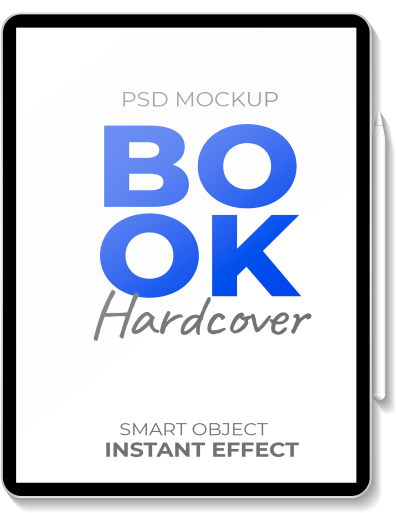
NEWSLETTER
Wpisz swój adres e-mail i zyskaj e-booka
Bez niechcianej poczty ani reklam
Tylko merytoryczne treści z obszaru digitalizacji produkcji
It is said that data is like new gold. It’s hard to disagree with that. After all, it is information that allows us to make more accurate decisions, better understand processes and predict what will happen next. But in practice, this gold is sometimes mixed. We collect data, store it, archive it in systems and spreadsheets – but do we really use it? Does any data analysis in business actually support decision-making?
In many organizations – especially manufacturing and service organizations – information ceases to be a tool and becomes an end in itself. Data is often collected because “that’s the way it needs to be,” “because the system requires it,” “because they will ask in an audit.”
The problem is that if we don’t know why such relevant data is collected, how it is analyzed and who is responsible for it – its practical value drops almost to zero. And this means measurable losses: time, money, people’s energy.
In today’s article, I’d like to address this topic more broadly by looking at the five most common reasons why data analytics in business fails to deliver real results. These are things I observe on a daily basis during implementations and consultations in industrial plants.
Importantly – many of these problems can be solved faster than it seems.
This is perhaps the most common problem that hinders effective data analysis in business. Each department in a company works a little bit in its own way. Different report formats, different systems, different approaches. Some work in Excel, others record data manually, someone else uses an MES system, and another keeps everything locally on their computer.
The lack of a uniform reporting standard results in duplication of the same information, errors and contradictions. Separate departments report the same events independently of each other, leading to disorganization and lack of confidence in the information being obtained.
What could be the solution to this problem? Build and implement a single, central source of truth. This could be, for example:
It is important that everyone works on the same basis and has access to the same information. Only then does business data analytics make sense.
Content that cannot be read, deciphered, or contains only residual information does not support business. They can even do harm. A classic example? Handwritten notes on paper reports, hard-to-read entries, lack of units, context, explanations. Often we see only raw values in the reports – e.g., “1000 pcs.” – but we don’t know if that’s right or wrong. There is no reference to a plan, an average, a target.
And after all, business data analysis is not only about collecting numbers, but above all also about interpreting them. Simple data analysis tools can help here, but especially indicators and dashboards that put the content in context. With the right data visualization, we know whether the result is in line with the norm or requires a response. The point is not to replace thinking with automatons – on the contrary. It’s about having a clear point of reference and being able to react faster.
Get 5 chapters of the book for free!
Join the newsletter and gain access to 40% of the book
“15 Steps to Buying an Information System“.
In many companies, data is nobody’s business. There is no one who cares about their quality, completeness and usability. When something is missing or wrong – no one feels responsible. And without an owner, it’s hard to talk about effective data analysis in business. Every process, every set of content should have a responsible person assigned to it. And no – it’s not just the IT department.
Production managers, quality leaders, operational specialists should also be responsible for data quality. A data owner is someone who knows why the data is collected, how it is used and who uses it. Without this, any analysis becomes movement in the dark.
Sometimes the data is available, but no one uses it – because simply no one trusts it. Too many inaccuracies, errors, ambiguities, versions of reports. As a result, instead of analyzing, people ask each other “how it really was”. And that, after all, is not the point.
Lack of trust means that hard information ceases to be the basis for decisions. Meanwhile, business data analysisshould be based on facts, not assumptions. If reports are disputed or everyone interprets them differently – the system loses its meaning. What can be done First of all, take care:
Reports should be up-to-date and understandable, so that they can be treated as a reliable source of information. Only then can we talk about effective data analysis.
Another problem is when the data is there – but nothing comes out of it. We collect information, store it, but don’t draw conclusions. There is a lack of tools, competencies and processes to translate them into decisions. It’s a bit like having a refrigerator full of ingredients, but no idea of any recipe.
Meanwhile, business data analysis should act like a good cook – show you what to combine with what to get the result. This is where the introduction of business intelligence tools comes into play. These are systems that themselves indicate anomalies, trends, deviations. Thanks to them, you don’t need to analyze every record – just look at the dashboard and you immediately know what needs our attention.
In the end, it’s worth saying it plainly: data by itself will not change anything. It’s not the numbers that generate value, it’s the decisions we make based on them. Without clear processes, owners and tools, data analytics in business will remain an empty buzzword. You don’t need to implement advanced BI or hire a team of business analysts right away.
Sometimes all it takes is a few small changes – designating responsible people, simplifying reporting, standardizing data sources. If you see that your data is not working for the company – start with these five points. Review them with your team. See what can be improved. Sometimes all it takes is a conversation to start a process that, over time, will evolve into a viable analytics culture.
Effective methods of analyzing and processing data can significantly contribute to improving the quality of operations, as well as productivity. They will also significantly support decision-making processes. It is also often the first step to better management, cost savings and growth – without wasting the potential we have at our fingertips.
If you’re interested in the topics of effective data management and analysis, check out the latest episode of Digitalizuj.pl videopodcast!
In it, you’ll learn why companies use only 20% of the information they collect, what are the most common barriers to analyzing operational data, and what can be done to actually support business decisions. I discuss specific examples, practical lessons and actions that can be implemented regardless of the level of digitization in an organization.